Telegraphing Financial Vortices: Your Financial Data is Trying to Tell You Something: Is Your Digital Wealth Management Process Built to Hear it?
by efrem on Wednesday July 19, 2017 at 1:19 pm EST | Tags: algos, bottom down analytics, Bubble, calculus, Chaos, Crash, crowd physics, Dragon-King, entropy, FinTech, fractals, gamma, gammarho, gauge geometry, intelligence, Investing, mania, motion, networks, Quantum, relational perception calculus, rho, tornadoes, trading, vortex, war gaming, Weath Management
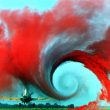
Telegraphing Financial Vortices: Your Financial Data is Trying to Tell You Something: Is Your Digital Wealth Management Process Built to Hear it?
The Fintech marketplace is in rapid flux; although we have a myriad of platforms for leveling the playing field between retail and institutional investors, they fall short at addressing the underlying physics of asset price movement and volatility behavior.
The existing way people think about how stock markets and asset prices migrate over time, is fundamentally flawed; so the founder, Efrem Hoffman, built a new class of technology to solve for this; centered on the mission of:
Helping Investors Generate Alpha and Protect their Assets by strategically “Investing in Times of Uncertainty” — Winning despite the unknown.
The strategy framework is formulated to go one step beyond AQR’s sniff test for sustainable alpha creation on five axes of merit, namely, it:
(i) produces an analytic record and walk-forward risk-return structure that indicates that the empirical investment edge is “persistent, pervasive, and robust across all asset classes and market regimes;”
(ii) offers an [ “explanation as to why and how the market inefficiency ( creating the opportunity ) comes into existence in the first place, and why it has not been and
(iii) continues to not be arbitraged away with evolving market pricing” ]; and goes one step further by:
(iv) requiring that there are no logical inconsistencies in the alpha-signaling logic across different fractal viewing scales that have overlapping position holding time-lines
By solving for the Quantum Measurement Uncertainty Enigma in the Financial Marketplace, Running Alpha goes beyond exploiting a simple risk premium based on a systematic behavioral bias or intuitive economic rationale, but leverages a long-held deeply entrenched philosophical model paradigm blind-spot that exploits the inability of conventional decision-makers ( both human and machine ) from:
anticipating an Known Unknown — ambiguity over factoring in and determining the market impact of our own buying and selling decisions, especially others reactions over several iterations of asset price formation;
and
acknowledging an Unknown Known — market players who naively dismiss that their actions can adversely work counter to their intentions;
(v) [“generates market positioning histories at the times of strategic opportunity ( entry and exit ), that have a well defined group of financial actors on the other side ( with limited arbitrage flows ) ] of the contemplated open market transaction.
Originally patented ( Patent #: 6,278,799 and 6,035,057 ) for Tracking down 3D Cloud Droplet Rotation Patterns preceding hostile Tornado events, it is now being successfully applied by the Toronto-Based Fintech Firm, Running Alpha, for addressing the need for efficiently generating Comfort-Adjusted Returns TM ( True Alpha — see NASADAQ Feature Article ) in world capital markets.
How? —
— By developing a new financial curve that re-casts and unifies the meaning of true alpha and beta; it’s called GammaRho.
Avoiding the curse of volatility variance ( winning in low-volatility environments and loosing in high volatility market conditions ) and making portfolios “anti-fragile” — recovering from draw-downs quickly and adapting their structure for higher levels of resilience and growth,
this new curve ( GammaRho ) was designed by the founder to quantify and understand the correlations among market sentiment perceptions at the tail — the way people and machines generate perceptions about the way they feel about the future when under elevated levels of stress and uncertainty.
What gives this curve a leg up on the prevailing view of observing market change, is that it’s about embracing the capacity for not only imagining the future, but for re-framing it and figuring out how to get from here ( a ) to there ( b ) along the paths of logical congruence and least action — by shaping the underlying structure of human and machine perceptions that ultimately create it (the future).
At first glance, when most people or machines look at a data set, they only observe how the market responded to the events of the day or history, and do not see the hidden variables or higher dimensional architecture of the data’s arrow of time —
— how the composition and network of market actors and their perception models of the world around them could have influenced non-local parts of the network to effect change differently ( along an alternate vector ), given a marginally different set of circumstances or economic, financial or geo-political stimuli.
To dive deep into this level of scenario analysis, we engineered the algorithms for peering into the cross-sectional time-delay uncertainties of the broad-band of measurement paradigms, that our adversaries may be using for analyzing data across different time-scales, asset classes, and geographies,
and
mathematically describing ( using network graphs ) how their perspectives are interlinked and entangled in a unified whole; even in situations where each market actor ( playing a passive or active role in order-book creation and price formation ) is making independent judgments and acting outside the crowd.
Where our competitors mostly live in the past, confined to either old precedents, extrapolating historic trends, and/or seeking to describe how things are — where the current value is and at what fundamental rate of growth a company is moving, or what technical momentum a stock is advancing, we play a different game;
Rather than see price moving through time, we render visibility of why things occur and how asset prices come into being.
By exploiting how nature solves problems and finds smart-cuts though quantum computing, we have found ways to unlock latent value in traditional and alternative data sets with limited history.
This is a big deal, because most of the newer data sources and alpha factors emerging today have a very short history and/or shelf-life.
By looking at the non-linear relationships among how financial particles ( decision-making perceptions and market order-flow ) reveal themselves to others ( the implied sentiment factor ) and the order book ( the explicit market factor ),
we have discovered new ways for quantifying the half-lives of news events, investor sentiment ( convictions and social-chatter ), and their asymmetric emotional effects on the direction and persistence of emergent price, volatility, and variance of volatility trends.
This matters, because it plays a key role in not only:
(i) knowing which assets to own — those offering the best edge in amplifying positive news and attenuating adverse outcomes, but also in
(ii ) shrinking the gap between when decisions are made and executed, resulting in a significant improvement in navigating investments around volatility and illiquid market environments, with reduced market friction, efficient execution, and favorable equity to draw-down performance.
In other words, whereas the traditional game of play has involved tracking the flow of money to extrapolate the the future path of price, to see what others cannot see, we look at the factors that power the migration of capital — namely:
the perceptions of influential decision-makers;
who they are ( investment capacity for each time-frame of investor ); and
where the decision-making audiences’ perception spaces are correlated across price levels and time.
This is important, because if you know where the people will be showing up, and which sandboxes they are going to be playing in, you can better identify and anticipate fund flows, instead of simply recording them in hindsight.
By shining light on previously dark regions of data ( Dark Data — the perception biases of the trader ecology ), Relational Perception Calculus (TM) is recasting the content of our existing data-store;
permitting us to resurrect data elements from the dust-bins of history, and see how they are behaving more like radioactive material, with a half-life that persists on into the future;
allowing “ancient” data to come alive and continue interacting with the changing market sentiment in new ways, much like relativity theory introduces reflexivity between gravity fields and mass.
So, it is not the prices that have memory, but the decision-makers ( people and robots ) that both re-enact and evolve their perceptions of what is happening and has happened in the past.
We keep track of these Decisions ( driven by previous perceptions ) that are yet to be implemented, based on prevailing liquidity conditions and the network feedback effects of both market-player perceptions and their reactions to changes in Perceived Value, Price and Volatility;
because without this new source of knowledge, we would be blind-sighted to abrupt regime transitions in market behavior; those “symmetry-smashing”events punctuated by asymmetric periods of:
positive feedback that amplify future perception biases; and
negative reflexivity conditions that yield extreme reversion events.
We make it our business to profit from both sides of this divide.
Laboratory results and real-life experiences, explains why the frequency and impact of unexpected ( surprise ) events are generally underestimated using previous model infrastructures
and
ontologies ( “sets of concepts and categories in a subject area or domain that shows their properties and the relations between them” )
— i.e. we are living in an age where 100 year floods are arriving every election cycle, or perhaps sometime soon, every earnings season.
We’re different because we don’t see information the way others do;
When we look at a data set, we see a living breathing organism that expels energy in the form of information to the environment ( sentiment context of marketplace);
rendering data more uncertain over time; thereby decreasing the value of insights that may have been learned and gleaned from previous trend recognition algorithms.
To get around this trap and impart meaning back into the less ordered ( entropy increasing ) data states, we add context by leveraging the increasing self-ordered structure of sentiment and perceptions biases driving them.
By measuring the pulse of where decisions are biased and being made, across a wide spectrum of market player viewing perspectives, instead of naively tracking outputs from the order-book in the form of previous transactions,
we can better identify and profit from discontinuties —
— i.e. sudden jumps and wild swings in value, such as opening market price gaps, that cannot be reliably anticipated with even today’s most sophisticated negative lag or leading indicators; since they all fall victim to quantum measurement uncertainty, as will be seen in the upcoming section) —
in market behavior that are not visible in the unfolding market data or fundamental information fields, that most all our competitors rely on as the only game in town.
By doing something others do not, we can better aim at extracting absolute alpha ( excess returns well above the market benchmark, without the use of any leverage) in all market environments ( Bull or Bear, High Volatility, Stressed, or directionless ), and economic and geo-political cycles.
Imagine a technology advancement so powerful that it can transform the way financial markets and economies work;
disrupting the way investors and entrepreneurs think about risk and uncertainty for better managing future expectations with the least amount of discomfort.
After 20 years in the making and several million dollars of R&D investment, it now exists, and its called Relational Perception Calculus ( RPC TM ).
Money is only one of the possible applications. But we started here, as it offers the longest and most reliable record of data on the digital assembly line for monetizing its insights.
To offer this new experience of wealth-building, we exploit a subtle, overlooked feature of quantum computing systems;
a secret sauce that helps us explain why records are made to be broken and diverge from past discourse, especially as they apply to profiting from and averting the episodic fits of panic, mania, greed, fear, and despondency —
— belonging to the rare 1% event class, which history repeatedly shows giving rise to the 99% of market pressures — information energy fields we, as investors, are otherwise collaterally exposed to each and every trading day.
As will be described in the proceeding section, this inconspicuous feature solves the problem of efficiently processing complex data and weighting and blending the factors ( unique technical / fundamental data fields ) of its many billions of interacting parts — human and machine based decisions.
To give you a sense of why this task has been a “wicked” challenge for the investment community, one must first understand its greater fundamental significance in the field of physics, namely:
Chaos Theory, and Quantum Uncertainty — expressed by the “Heisenberg Uncertainty Relation” in scientific circles.
First off, Chaos Theory ( “Deterministic Chaos” in scientific parlance ) simply means that extraordinary precision would be needed in measurements, in order to know what is going to happen in the long run — i.e. “Butterfly Effect” — something as appearing innocuous as a flap of a butterfly’s wings in the Canadian Rockies can cause global weather patterns to yield economic peril across the Atlantic.
In other words, sensitive dependence on initial conditions translates into widening divergences as time marches on, so much so, that what first starts out as an independent path in price, time, and value, quickly starts crossing others ( alternate paths of equally independent agents of market change ) in the future;
causing interactions at the crossroads to deem the initial extrapolation of past trends inaccurate across all fractal scales of human and machine observation.
Relational Perception Calculus renders high-definition visibility of these interactions ( in perception space ) before they show up in the macroscopic behavior of price, sentiment or fundamental economic or financial indicators.
Chaos Theory is the least of what should be keeping our quant competitors up at night;
Quantum Uncertainty is the Mammoth-Sized “Elephant in the Room.” It simply means that precise values for both position and momentum — velocity*mass ( transaction volume ) of a particle ( price or value ) are, in principle, unknowable.
In a sense these numbers don’t even exist until they are measured, because before they are observed they exist in more than one state at the same time;
until the final moments of decision-making, when the observer probes the region where the particles reside, and disturbs the configuration, tipping the system into a state that is radically different from what was intended to be measured.
Since all measurement devices, no matter how small interact with the environment; it renders conventional observation tool-sets ineffective at quantifying instantaneous change without introducing uncertainty;
and when combined with deterministic chaos, can profoundly influence even macroscopic ( i.e. macro-economic ) behavior in unintended ways. As will be seen later, our algorithms not only corrects for these episodes of collateral damage ( or beauty ), but leverages them against less informed traders for competitive positioning.
This type of ambiguous measurement goes on all the time in the financial and economic marketplace, and is known as “probing the markets.” Market players do this to get inside the head-space of their adversaries, and acquire access to their intentions;
it involves placing phantom orders ( orders of magnitude more devious and inconspicuous than spy shoppers in the brick and mortar economy ) — orders lacking deliberate execution.
This type of “spoofing” was prominently displayed during the flash crash of 2010 by market predators, to game the system for short term reward and long-term strategic advantage.
Our system flagged this nefarious behavior to within a three hour orb ( window ) over one month before its onset, and continues to actively monitor such activities to circumvent future portfolio landmines.
To this end, today’s robo-traders, no matter how sophisticated, relying for the most part on Artificial Intelligent (AI ) machines, that learn from experience ( real-world measurements ), are doomed to fall prey to this uncertainty and ever-increasing disorder ( High Entropy market condition ).
While the laws of physics may inherently prevent us from observing the physical universe without ambiguity, we have created a RPC (TM) tool-set that allows our in-house traders and venture partners for the first time to gain insight into their competitors perception space without the need for probing or measuring price and momentum in real-time.
Our proprietary quantum decision-making metrics allow us to calibrate fields of forward price ( yet to be actualized in the future — we call this Gamma ) with the specific values of zero momentum balance points ( we call this Rho ), spanning across all investable time scales, telescoping upwards from the sub-millisecond interval of high frequency traders to the time-lines of multi-generational investors.
This GammaRho curve enables our investment algorithms to not only provide a layer of transparency that our peers consistently lack, but allows us to overcome the cost of: being human; or running algos, whose most subtle measurements impart collateral uncertainty into the observed market outcomes.
The problem is “not whether buy and hold works in theory; it does not work on average in practice,” because people who make investment decisions, and the people who use machines relying on ground-based risk intelligence, do not have the fortitude to resist media chatter and market noise ( i.e. data exhaust — redundant data and statistical information mirages ), during periods of elevated uncertainty.
What investors really need is to raise their “Emotional Alpha” IQ —
— the best returns they can achieve for the level of stress per unit of risk ( and unintended uncertainty ) they’re going to have to take on for the lifetime of their investment.
Our algo factors in how much discomfort each investor type is likely to endure from not knowing the future state with certainty.
This pain often attacks investors at two points —
(i) when investors panic out of positions after a sudden market setback;
or
(ii) during times of heightened uncertainty surrounding economic conditions, when people or machines either:
(a) freeze like deer just before the onset of a large informed market order — that precipitates a cascading sequence of orders that unwind the excesses of a crowded trade in the form of a market crash; or
(b) make the all too frequent mistake of exiting positions too early, just before a large market advance — compressed over a relatively short time interval — accounts for the majority of the long term gains.
Running Alpha solves for both of these pain-points, building portfolios with high levels of Comfort-Adjusted Returns (TM).
With a keen focus on these fronts — Profiting from Disruptive Uncertainties in our Volatile Planet (TM), the company’s founder, Efrem Hoffman has been building Social Traction for Running Alpha’s technology, from:
Features on the March 22nd, 2016+ edition of DailyFintech ( a Swiss-based Fintech Conversation and Thought-Leadership Hub ) as:
One of the 10 Emerging FinTech platform leaders highlighted “in the AI business of “sniffing out” [signals] in digital wealth management, and changing the value proposition of financial analysts and asset managers” ; to
Nominations for the 2016 Benzinga (BZ) Fintech Awards, where RunningAlpha placed as a Finding Alpha Finalist — at a New York City Gala held at the Tribeca 360 on May 24th, 2016.
In the DailyFintech article, references are made to alternative solutions that “can warn you before the market undergoes a nasty selloff.” Although these platforms use real-time data from exchanges —
“[ looking for patterns and searching for clusters of traders who are bailing out of an investment and providing clear visual signals when sellers are gaining strength before a broad slide kicks in”] —
The Running Alpha methodology goes beyond analyzing visible data, which can be gamed and trolled by your market adversaries.*
The founder was recently Interviewed by Benzinga News for an Exclusive NASDAQ.com Feature Article on this technology, which was released on May 10th, 2017.
Mr. Hoffman also served/serves as an invited keynote speaker / debate panelist at live Bloomberg Charts Day, Terrapin Quant World Canada 2017 and Trading Show Chicago 2017, TradersWorld Expo, and Toronto Hedge Fund Hotel Event(s); and
his thought-leadership and insights have been numerously quoted in the international press — i.e. CFA Magazine, NASDAQ news, Huffington Post, Esquire, Hamburg News, BloombergView, WSJ, CNBC breaking article relating to Trump Market Factor, and
other digital media sources, from an exclusive feature article in a Best-Selling Amazon Book, authored by the owner of TradersWorld Magazine,
highlighting the best practices of among top traders and trading analysts, to upcoming feature articles in e-books of leading digital media companies — set to hit the wire this fall, 2017.
The NASDAQ articles showcases how Running Alpha, a Toronto-Based FinTech firm, is redefining the meaning of information and unlocking new insights into asymmetric sentiment-biases, for
building smart portfolios that can hedge-for-a-profit instead of a cost against global market uncertainty and tail-risk events.
To address these issues, Running Alpha created the RPC Mathematical Physics Tool Set (TM) —
— a systematic algorithm and set of visual information displays; unifying, for the first time, the dynamics describing the global and local networking interaction effects among human perceptions, and the machine paradigms and behaviors executing on them;
serving as a self-generative time series analysis tool for working out the equations of motion — by finding smart-cuts around performing an exponential number of calculations distributively in real-time.
This is not only leading to better predictions of the movement of people, capital, and ideas; but is also:
putting boundaries around future events, namely, the range of values in the price spectrum that will manifest in the next incarnation of marketplace interactions — that our competitors only see later.
Powered by:
Running Alpha’s Revolutionary Multi-Channel Relational Calculus Approach — lying at the intersections of Quantum Decision Gaming, Gauge Geometry, Mathematical Physics, Cognitive Science, Market Reflexivity and Trader Ecology, Quantum Biology, Chaos Theory, Dragon-King Analysis ( analysis and prediction of factors underlying super-exponential growth in complex dynamic systems), Information/Communication Theory, Wavelet & Multi-Fractal Analysis, and Machine Intelligence),
the founder has been hard at work around the clock over the past two decades collaborating with world-class practitioners, academics, and entrepreneurs; building agile asset pricing solutions through testing and iterating on the next generation of core technology in both the laboratory and real-life setting.
NEXT STEPS
The founder is also currently in the works of packaging up the core innovation into consumer-oriented products and services for some of his retail and institutional contacts, that have expressed deep interest in Running Alpha’s Alternative Reporting and New Category of Sentiment-Aware and Perception-Inspired Passive Smart-Beta Indexing Strategies.
To learn about how you can license Relational Perception Calculus (TM)Portfolio Indexing and Active Strategies to augment your Intelligence,
Start connecting today with the research framework architect, Efrem Hoffman on LinkedIn.
About the Founder — Efrem Hoffman
With over 20 years experience commercializing advanced technologies out of the physics lab and into the trading room, for:
(i) helping professional value managers and angel investors find smarter investments in the face of volatile market conditions; and
(ii) persistently profit from both this volatility and their mis-pricing — arising from the failure of the prevailing best practices to track and quantify the extreme uncertainty of market variables imparted by our volatile planet,
the founder is passionate about employing this technology for augmenting HUMAN INTEL on connecting the dots; and bringing together insights about markets, macro policy and geopolitics, that signal important investable, and emerging trends in world equity, commodity, and financial futures and currency markets.
Tapping into this collective wisdom, Efrem is passionate about rigorously and routinely stress-testing the research framework in the midst of financial tornadoes; with a record of successfully warning investors of the demise of WorldCom, Bear Stearns, Lehman Brothers, GM, among others, before any signs of trouble emerged in 2008. On-balance, a review of the much more mixed market settings, scrolling back as far as 400 years across world capital markets, shows similar predictive intelligence.
With uncanny accuracy and specificity, his market calls were used to help financial traders successfully prepare ahead of other notable capital market hurricanes, including”
the Dot.Com Bust, 2005-07 USA Housing Crash, as well as the subsequent re-acceleration of growth that followed the Great Recession ( particularly from 2013 ), and that continues to fuel the USA-focused market rally today.
Our current Crisis Surveillance Alert System has recently issued a warning for its abrupt end, at least in the near to mid term. This market call was recently picked up by the financial media and put on record in a feature market brief by Benzinga TV | Pre-Market Prep Radio on June 29th, 2017 ( segment starts after 16:19 minutes of play ).
Most notable among his Tail-Risk pre-casts of wild sentiment jet-stream behavior, was the flash crash of May 2006 — accurately telegraphing in magnitude over two months in advance, while striking inside the exact hourly timing window of the anticipated peak tail-risk threat.
The Bottom Line to Efrem’s On Going Research is:
- The events we perceive as ordinary every-day occurrences are actually born out of the background noise of prior crises and unusual market aberrations.
- Thus by focusing on the rare 1% Outliers rather than eliminating them, the agenda is to gain clarity on the 99% of market pressures that influence decisions each trading day!
- New Perceived Risk & Capital Asset Valuation Framework, founded on: Long and & Short Leadership Rotation of Asset Class Rankings delivering:
- Portable, Low-Cost Tail-Risk Solutions for Professional Managers looking to:
- Hedge-For-A-Profit instead of a cost in All-Volatility Environments with Absolute Alpha.
